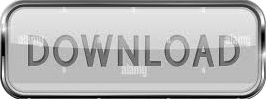

Proceedings of the Twenty-Ninth AAAI Conference on Artificial Intelligence: 2267–2273. Recurrent convolutional neural network for text classification. Meeting on Association for Computational Linguistics: 423–430. Bittersweet! Understanding and managing electronic word of mouth.
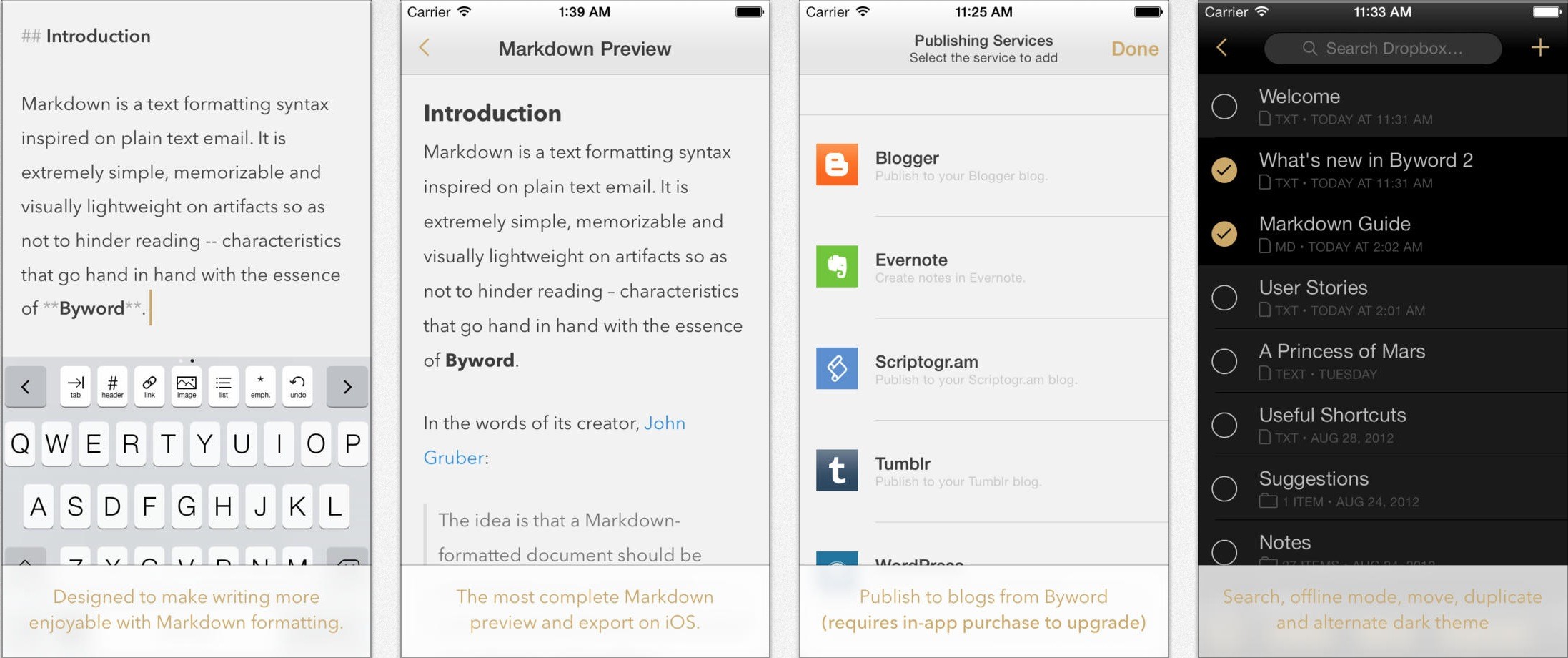
International Conference on Web Search and Data Mining, ACM. Reducing the dimensionality of data with neural networks. Journal of Systems Science and Systems Engineering 27(5):542–558. Products ranking through aspect-based sentiment analysis of online heterogeneous reviews. Proceedings of the EACL 2012 Workshop on Computational Approaches to Deception Detection: 23–27. In search of a gold standard in studies of deception. Gokhman S, Hancock J, Prabhu P, Ott M, Cardie C (2012). A synopsis of linguistic theory 1930–1955. International Joint Conference on Natural Language Processing: 14–18.įirth J R (1957). Detecting deceptive opinions with profile compatibility. Syntactic stylometry for deception detection.

Journal of Machine Learning Research 12(1):2493–2537.įeng S, Banerjee R, Choi Y (2012). Natural language processing (almost) from scratch. Journal of Parallel & Distributed Computing: 160–167.Ĭollobert R, Weston J, Bottou L, Karlen M., Kavukcuoglu K, Kuksa P (2011). A unified architecture for natural language processing: Deep neural networks with multitask learning. Proceedings of the Twenty-Second International Joint Conference on Artificial Intelligence: 1237–1242.Ĭollobert R, Weston J (2008). Flexible, high performance convolutional neural networks for image classification. Knowledge-Based Systems 50(3):44–59.Ĭiresan D C, Meier U, Masci J, Gambardella L M, Schmidhuber (2011). Preference-based clustering reviews for augmenting e-commerce recommendation. Journal of Systems Science and Systems Engineering 27(6):709–726.Ĭhen L, Wang F (2013). An empirical feasibility study of societal risk classification toward BBS posts. Do consumers use them? Proceedings of Conference on Association for Consumer Research: 129–134.Ĭhen J, Zhou X, Tang X (2018). Journal of Systems Science and Systems Engineering 23(2):212–230.Ĭhatterjeei P (2001). Topics and trends of the online public concerns based on Tianya forum. The outcome of this study provides potential implications for online review management and business intelligence of deceptive review identification.Ĭao L, Tang X, (2014). Moreover, the DCWord-M strategy outperforms the DCWord-A strategy in review representation for deceptive review identification. Experimental results on the Spam dataset and the Deception dataset demonstrate that the DCWord-M representation with LR (Logistic Regression) produces the best performances and outperforms state-of-the-art techniques on deceptive review identification. The average-pooling strategy (called DCWord-A) and max-pooling strategy (called DCWord-M) are used to produce review vectors from word vectors. Unlike state-of-the-art techniques in seeking best linguistic features for representation, we use word vectors to characterize contextual information of words in deceptive and truthful reviews automatically. The basic idea is that since deceptive reviews and truthful reviews are composed by writers without and with real experience on using the online purchased goods or services, there should be different contextual information of words between them. This paper proposes a deep learning approach for text representation called DCWord (Deep Context representation by Word vectors) to deceptive review identification. Due to the anonymous and free-for-all characteristics of online forums, it is very hard for human beings to differentiate deceptive reviews from truthful reviews.
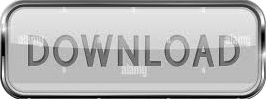